According to Forbes, predictive analytics can improve processes using machine learning and historical data like weather patterns, consumer behavior, and gas price fluctuations. Predictive analysis leverages AI and algorithms.
One of the key benefits of predictive analysis in advertising is its ability to identify patterns and trends that may not be immediately apparent. For example, predictive analysis can help advertisers identify which channels and platforms are most effective for reaching specific audiences, which messages resonate with different segments, and which campaigns are most likely to drive conversions.
In this article, we will examine the meaning of predictive analysis and how it can be helpful for outdoor advertisers.
What is Predictive Analysis?
Predictive analysis is a technique used to analyze large amounts of data and predict future trends and behaviors. Using historical data and statistical modeling, predictive analysis can help advertisers understand customer behavior and preferences, inform advertising strategies, and improve targeting efforts.
Predictive analysis is critical because it gives accurate predictions based on past data. In outdoor advertising, PA can help optimize advertising spend and improve ROI. Advertisers use PA to identify high-value customers and target them with appropriate messages.
It also helps identify customer segments based on demographic, behavioral, and psychographic data. Advertisers can then tailor their marketing campaigns to each segment’s preferences, increasing the likelihood of engagement and conversion.
Furthermore, predictive analysis enables advertisers to identify the best locations and times for placing outdoor ads. Advertisers can identify high-traffic areas, peak times, and relevant events that align with their target audience by analyzing historical data on foot traffic, demographic information, and contextual factors. This ensures maximum visibility and impact for their ads.
Key components and techniques used in predictive analysis
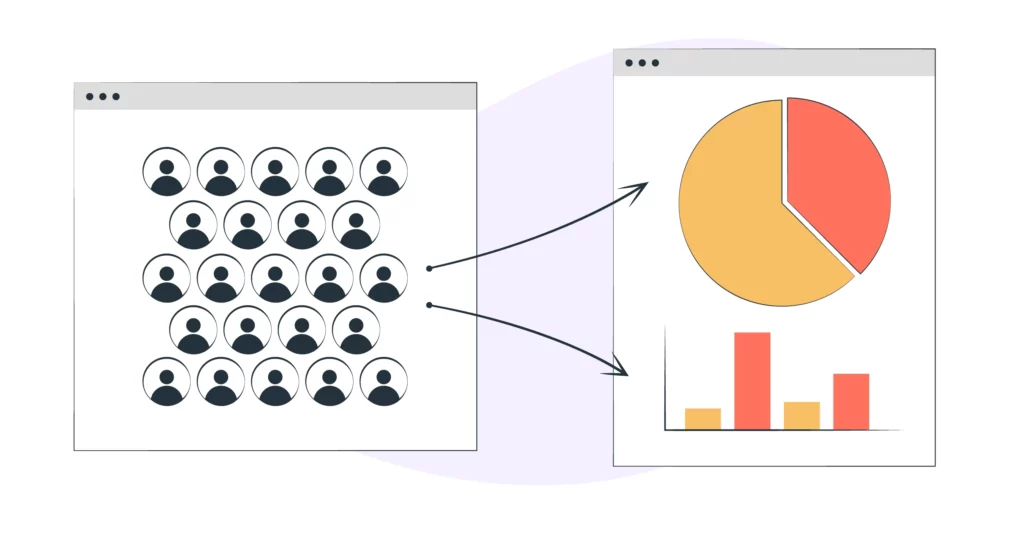
In predictive analysis, several key components and techniques are used to extract insights and make accurate predictions. Here are some of the essential components and techniques:
Data collection from multiple sources:
This is the first technique used in PA, and it involves gathering relevant and high-quality data from various sources, including databases, surveys, sensors, social media platforms, and other data repositories.
Data cleansing and preprocessing:
After collecting the data, it will be cleaned and preprocessed before it is analyzed. This step involves handling missing values, removing duplicates, correcting errors, and transforming data into a consistent format. Preprocessing techniques may also include normalization, standardization, and feature scaling.
Feature selection and engineering:
Feature selection involves identifying the most relevant variables or features from the dataset that significantly contribute to the prediction task. Feature engineering involves creating new features or transforming existing ones to improve the predictive power of the models. Techniques like dimensionality reduction, correlation analysis, and domain knowledge are employed for effective feature selection and engineering.
Exploratory Data Analysis (EDA):
EDA involves visualizing and analyzing the data to gain insights, identify patterns, and understand relationships between variables. Techniques such as histograms, scatter plots, box plots, and correlation matrices are used to explore the data and uncover initial insights.
Model selection, training, and evaluation:
The model choice depends on the nature of the data and the specific prediction task. Common models used in predictive analysis include linear regression, logistic regression, decision trees, support vector machines, and neural networks.
The selected model is trained using historical data to learn patterns and relationships. The trained model is then evaluated using validation or testing datasets to assess its performance and determine its predictive accuracy. Evaluation metrics such as accuracy, precision, recall, F1-score, and ROC curves are used to measure model performance.
Model deployment and monitoring:
Once a predictive model is trained and evaluated, it can be deployed in a production environment. The model continuously monitors new incoming data and provides predictions or recommendations in real-time. Regular monitoring of the model’s performance ensures that it remains accurate and up-to-date.
These key components play an essential role in conducting an effective predictive analysis. As an outdoor advertiser, you must carefully select some of the appropriate predictive models for outdoor advertising.
Selecting appropriate predictive models for outdoor advertising
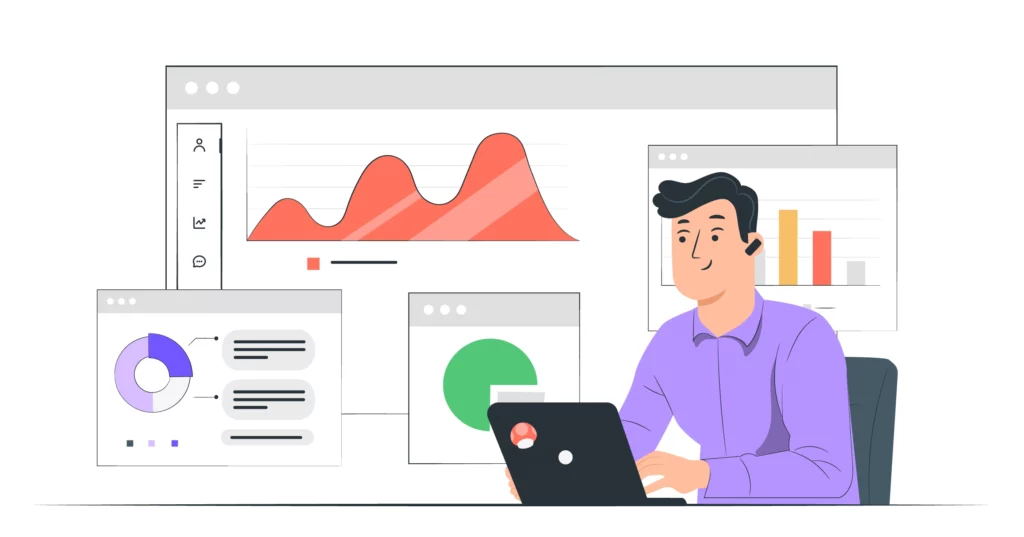
Selecting the appropriate predictive models for outdoor advertising involves considering various factors, such as the nature of the data, the prediction task, and the desired level of accuracy. Here are a few commonly used predictive models for outdoor advertising:
Regression Models:
Regression models are used when the prediction task involves estimating a numerical value, such as forecasting the number of impressions or predicting ad performance metrics like click-through rate (CTR) or conversion rate. Linear, polynomial, and multiple regression are common regression techniques used in outdoor advertising.
Classification Models:
Classification models are suitable when the prediction task involves categorizing or classifying data. For example, predicting whether a customer will respond to an outdoor ad or not. Popular classification algorithms include logistic regression, decision trees, random forests, and support vector machines (SVM).
Time Series Models:
Time series models are designed to analyze and predict data that evolves over time. They are useful for forecasting trends and seasonality patterns in outdoor advertising, such as predicting foot traffic or ad performance over different periods. Popular time series models include ARIMA (Autoregressive Integrated Moving Average), exponential smoothing models, and recurrent neural networks (RNNs).
Clustering Models:
Clustering models are employed when the goal is to identify distinct groups or segments within the target audience. This helps with targeted ad placement and personalized messaging. K-means clustering, hierarchical clustering, and Gaussian mixture models are commonly used clustering algorithms.
Ensemble Models:
Ensemble models combine multiple individual models to improve predictive accuracy. Techniques such as bagging, boosting, and stacking create ensembles. Ensemble models like random forests and gradient boosting machines (GBMs) are often applied in outdoor advertising to enhance the robustness and accuracy of predictions.
The selection of the appropriate model depends on the specific requirements of the prediction task, the available data, and the desired level of interpretability and the predictive power. It is essential to evaluate different models, compare their performance using appropriate metrics, and choose the model that best fits the problem.
Benefits of Predictive Analysis in Outdoor Advertising
PA can be beneficial to outdoor advertising. Here are some of the benefits:
- Helps segment the target audience based on demographics, behavior, and preference.
- Helps in analyzing optimal locations for ad placement.
- Predict consumer behavior and responses to outdoor ads.
- Forecast the performance of outdoor advertising campaigns.
- Used to analyze and predict competitors’ advertising strategies and effectiveness.
Conclusion
Predictive analysis is a great tool for forecasting future happenings based on past data. PA will continue to thrive with the growing rate of AI and algorithms.
However, in outdoor advertising, predictive analysis can help advertisers to measure how their target audience will receive and react to their campaigns. This makes PA models important for an effective ad campaign.
At Nickelytics, we understand the power of machine learning and AI for data collection and analysis. Our accurate location data makes targeting precise and helps predict the impact of your campaign.